GLH QC Smart-Cart
Quality Automated
Automated Liquid Handler QC Excellence
The GLH QC Smart Cart is a semi-to-fully automated machine that can move from station-to-station and provide application support of liquid handler processes and quality control.
- Streamline the process of Quality Control
- Improve testing performance
- Maximize operational readiness
- Automated and optimize scheduled testing cycles during times of incubation or down times or in parallel with other processes
- Enable integration with islands of automation
- ALH and Volume Verification Instrument agnostic
Addressing Industry Challenges
Historically, quality control systems relied on manual testing by technicians, which introduced human error and required specialized knowledge. While robotic arms have replaced manual pipetting in many applications, they have not adequately addressed testing liquid handlers. The GLH QC Smart-Cart bridges this gap by offering:
- Versatile Volume Verification: Supports single-dye, dual-dye, fluorescence, and gravimetric methods, tailored for multi-channel testing, environmental variability, and scalability.
- AI-Driven Optimization: Uses artificial intelligence (AI) and machine learning (ML) algorithms to evaluate performance and recommend adjustments, ensuring consistent and reliable results.
- On-Deck and Off-Deck Testing: Features a flexible design for volumetric verification and calibration directly on or off the deck of automated liquid handlers (ALHs).
Innovative Features and Functionality
The GLH QC Smart-Cart integrates seamlessly with diverse laboratory environments. Key features include:
- Modular Design: Compatible with a wide range of liquid handler robot decks, robotic arms, grippers, readers, and microplate scales.
- Open Communication Standards: Utilizes SILA for interoperability among laboratory instruments, with support for additional programming protocols.
- Performance Testing Library: A comprehensive database of testing methods to control and optimize various liquid handlers and absorbance readers.
- Predictive Maintenance: Learning algorithms assess and recommend maintenance schedules, minimizing downtime and maximizing operational efficiency.
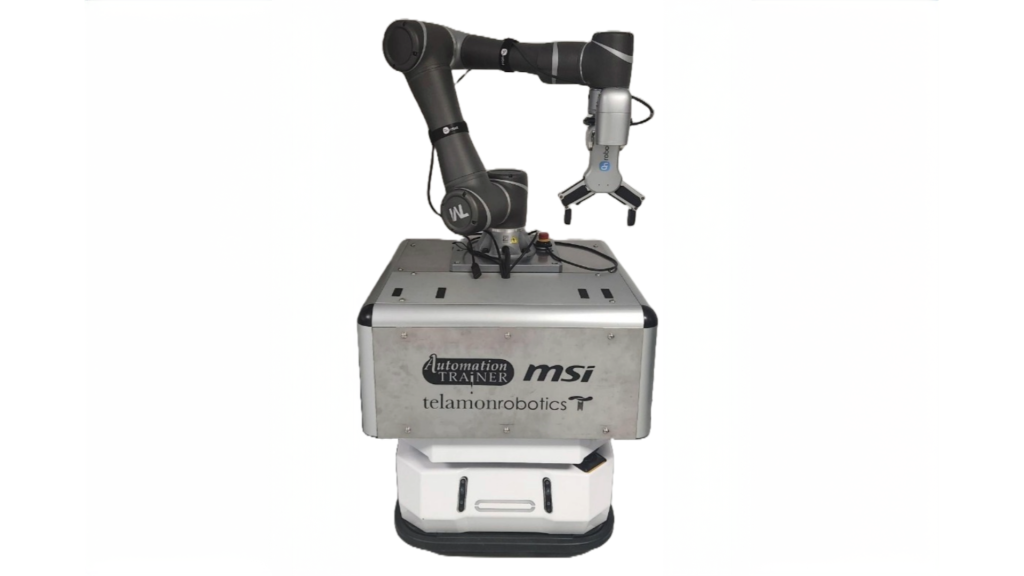